What Are the 4 Stages of an AI Workflow?
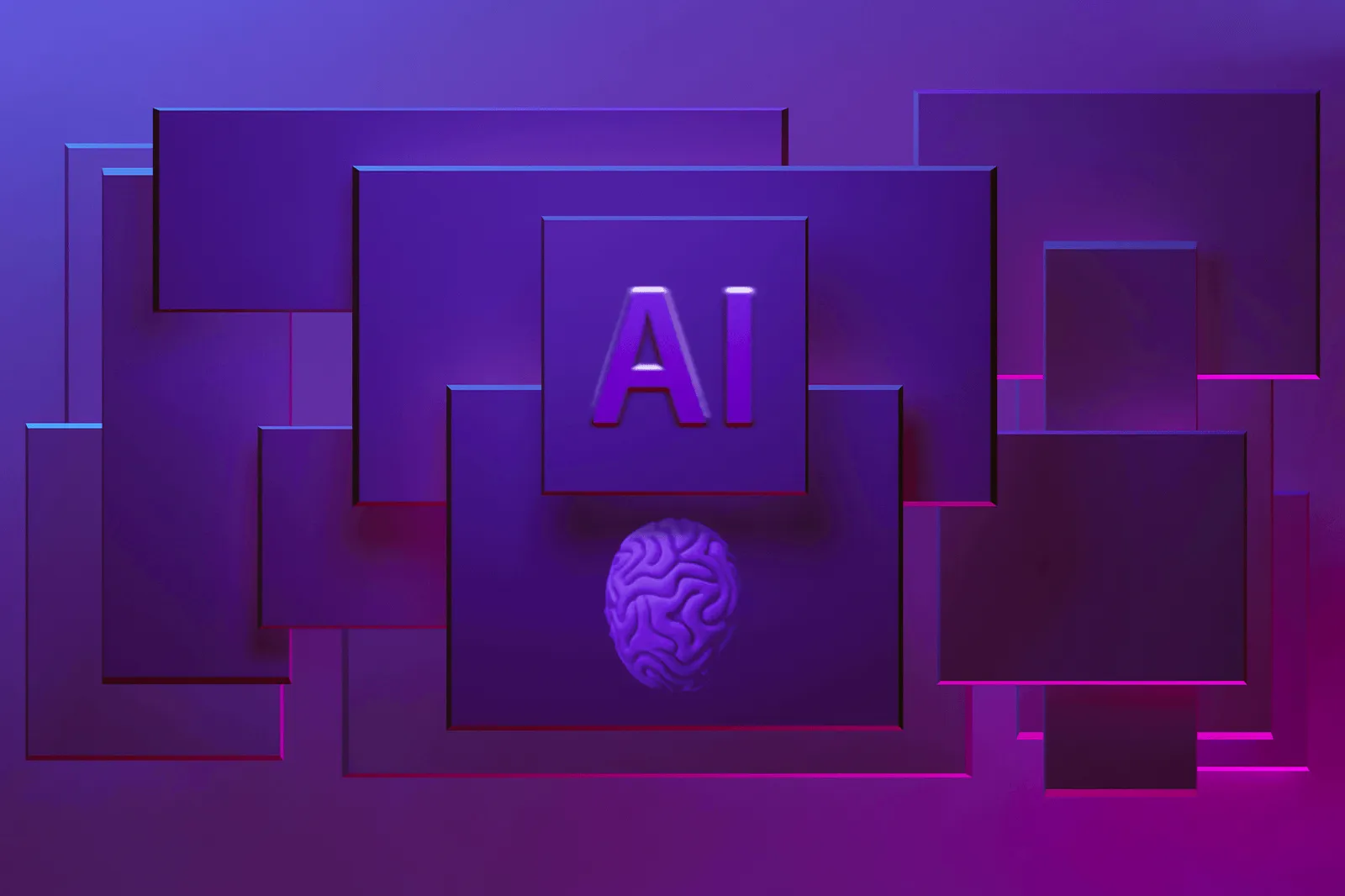
AI workflows, despite their variety and applications, generally follow four fundamental stages that ensure a systematic and efficient execution of tasks. These stages act as the backbone for any AI-driven process, helping transform raw data into actionable insights or automated solutions. From automating complex processes to scaling content production, understanding these steps is key to leveraging AI effectively.
This article breaks down the four core stages of an AI workflow, illustrating how they apply across diverse use cases. Whether you’re optimizing SEO strategies, creating content, or analyzing data, these stages provide a universal framework to guide AI implementations.
What is an AI Workflow?
An AI workflow is a structured process that guides the execution of AI-driven tasks from start to finish. It involves a sequence of steps, typically including data input, processing, analysis, and output generation, all powered by artificial intelligence. AI workflows are designed to automate repetitive tasks, improve decision-making, and streamline complex processes.
They can be customized to suit specific use cases, such as content creation, data analysis, or predictive modeling. By integrating AI workflows into their operations, businesses can achieve greater efficiency, scalability, and accuracy, enabling them to focus on strategic objectives and deliver consistent, high-quality results.
The goal of an AI content workflow is to systematically generate and refine high-quality, relevant content that aligns with the brand tone, resonates with the target audience, and supports SEO objectives. By leveraging tools like AirOps, organizations can automate content generation, optimize content for specific keywords, and create personalized, data-driven experiences across multiple platforms.
4 Stages of an AI Workflow
Now that we’ve established what an AI workflow is, here are the four steps each AI workflow takes to complete its specific task.
1. Data Collection
Data collection is the foundation of any AI workflow. In this step, raw data is gathered from various sources, such as databases, APIs, sensors, or user interactions, depending on the application. The quality and relevance of the collected data are critical, as they directly impact the performance of the AI model.
Data can be structured, like numerical values or tables, or unstructured, like text, images, or videos. Tools and systems are often employed to automate this process, ensuring efficiency and scalability. For example, in content generation workflows, data such as keywords or user preferences is collected to guide the output. By aggregating comprehensive and accurate datasets, this step sets the stage for effective AI operations.
2. Data Processing
Once data is collected, it undergoes processing to prepare it for analysis and decision-making. This step includes cleaning (removing duplicates, fixing errors), normalization (ensuring consistency), and transformation (converting raw data into a usable format). AI models require structured and well-organized input to deliver accurate results.
For instance, in an AI-driven SEO workflow, raw keywords may be processed into clusters or paired with supporting metrics like search volume. Advanced tools and algorithms automate much of the data processing, reducing manual effort and ensuring consistency. A well-executed data processing phase ensures the AI workflow runs smoothly and delivers reliable results.
3. Decision-Making and Training
In this stage, the AI system analyzes processed data to identify patterns, make predictions, or derive insights. This may involve applying pre-trained models or training new models using machine learning algorithms. Decision-making is often iterative, where models learn from previous outcomes to improve accuracy over time.
For instance, in content optimization, an AI tool might decide on the best keywords or internal links based on historical performance. This step also includes validating the model’s decisions against benchmarks or business objectives. By continuously learning and refining, the AI workflow becomes more effective and aligned with its intended purpose.
4. Action Execution
The final step is executing the decisions made by the AI system. This could involve generating outputs, triggering automated processes, or delivering actionable insights. For example, in a programmatic SEO workflow, the AI might publish optimized pages directly to a CMS or generate a detailed report for human review.
Action execution ensures that the insights or predictions from the workflow translate into tangible results. This phase often includes monitoring and feedback loops to track performance and identify areas for improvement. By automating execution, AI workflows save time, reduce errors, increase traffic, and allow teams to focus on strategic tasks.
Benefits of Implementing an AI Workflow
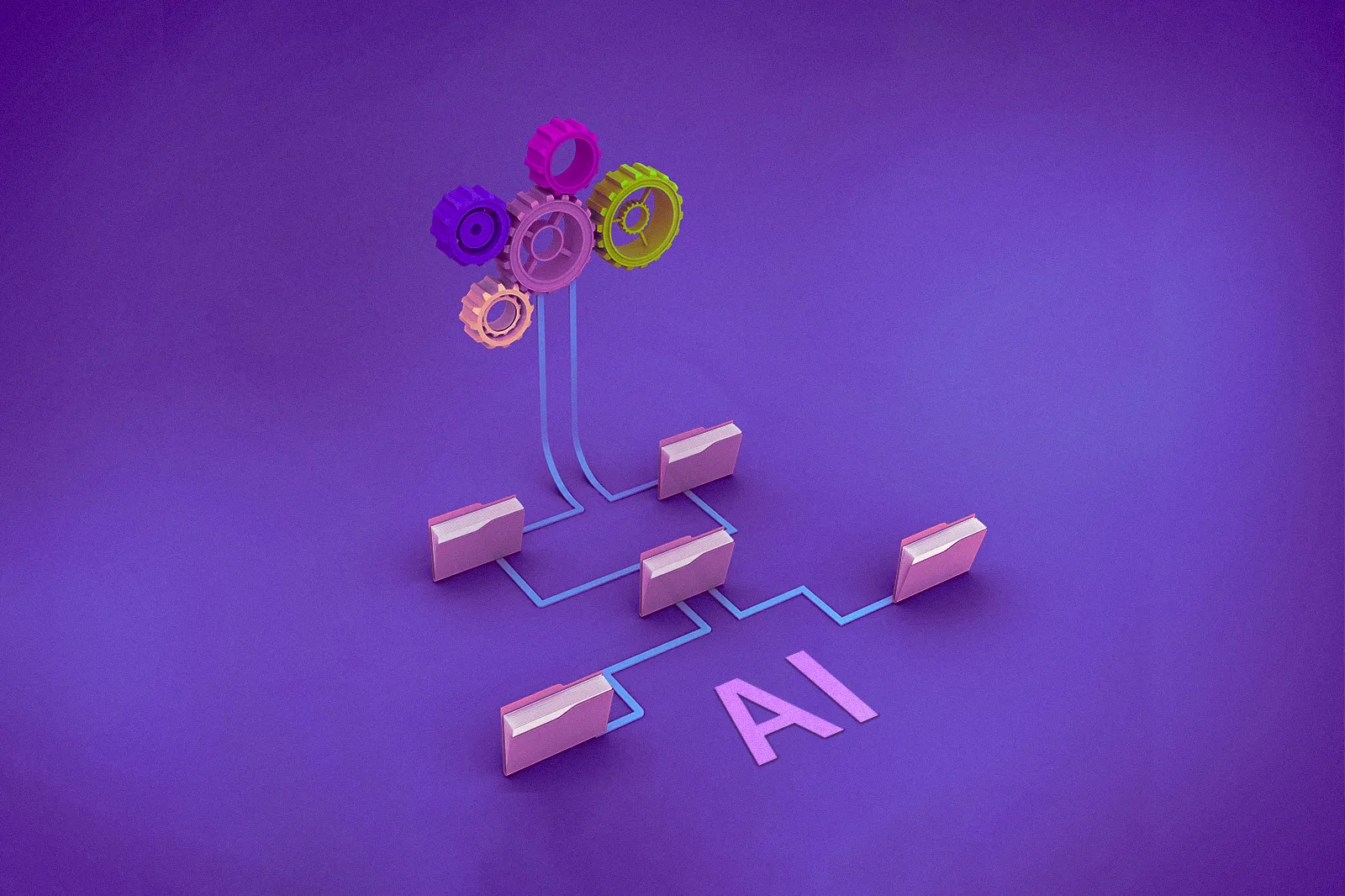
Implementing an AI workflow in content operations provides transformative advantages for organizations aiming to scale and optimize their content creation processes.
Here are the key benefits that come with adopting a structured AI approach to website content management.
Increased Success Rate
Following a well-defined AI workflow significantly improves the chances of project success. By ensuring that each necessary step in the AI development process is meticulously followed, you can minimize the risk of overlooking critical aspects and increase the likelihood of achieving your desired outcomes.
Risk Reduction
An AI workflow in content operations identifies potential issues, such as inconsistent messaging or SEO misalignment, early in the process. By addressing these challenges proactively during the planning and optimization phases, organizations can reduce the risk of publishing ineffective or poorly performing content. This proactive risk management protects brand reputation and maximizes return on investment.
Improved Efficiency and Productivity
Adopting an AI workflow streamlines the entire content lifecycle, from research and creation to distribution and analysis. With clearly defined stages, content teams can collaborate more effectively, avoid duplicative efforts, and accelerate production timelines. Automation tools integrated into the workflow handle repetitive tasks, allowing teams to focus on high-value activities like strategy and storytelling, boosting overall productivity.
Enhanced Quality of Content
Implementing an AI-driven workflow ensures that content undergoes rigorous refinement at each stage. AI models assist in optimizing headlines, improving readability, and tailoring content to specific audience segments. Continuous feedback loops help maintain quality standards and adapt content to evolving audience preferences. Post-publication analytics and AI tools ensure that content continues to perform well over time, creating sustainable value for the organization.
What Are the Challenges in Implementing an AI Workflow?
Implementing an AI workflow is not without its challenges. Here are some challenges organizations face when adopting AI and how to overcome them.
Poor Data Quality
The quality of your AI outputs heavily depends on the quality of your input data. Unstructured, unformatted, or insufficient data can significantly hinder the performance of your AI models.
To address this challenge, you need to establish robust data governance practices. This includes cleansing and organizing your data into standardized formats, ensuring consistency and compatibility with your AI systems. Generating AI content requires high-quality data to produce accurate and relevant results.
Lack of Infrastructure
Running AI workflows efficiently demands high-performance specialized infrastructure. Legacy systems may not have the necessary power density or cooling capabilities to support AI workloads.
Investing in the right hardware and infrastructure is key to successful AI implementation. Consider upgrading your systems or exploring cloud-based solutions that offer scalable resources tailored for AI workflows.
Soaring Costs and Complex Operations
Implementing AI can be a costly endeavor, involving expenses in development, infrastructure, and hardware. Organizations need to carefully assess their AI investments and ensure alignment with business goals.
Managing AI workflows also introduces complexity in operations. It requires expertise in data management, interconnectivity, and team coordination. Assembling a skilled team with the right mix of technical and domain knowledge is vital for navigating the intricacies of AI workflows.
To mitigate costs and streamline operations, consider leveraging AI platforms and tools that provide pre-built models, automation capabilities, and intuitive interfaces. These solutions can help you accelerate AI development, reduce manual efforts, and optimize resource utilization.
How to Build an AI Workflow?
Building an AI workflow requires the right tools to streamline processes, ensure accuracy, and align outcomes with your business goals. With AirOps, creating AI workflows becomes intuitive and scalable, empowering businesses to automate complex tasks and optimize operations.
AirOps provides a powerful drag-and-drop workflow builder that allows you to design custom AI workflows tailored to your specific needs. Whether it’s automating content creation, improving SEO strategies, or analyzing large datasets, AirOps simplifies each step. Begin by defining the data inputs—such as keywords, user preferences, or datasets—using integrated tools like Google Search Console or Semrush for seamless imports.
Next, configure the processing phase. AirOps enables you to clean, organize, and structure data effortlessly. The platform also supports using Brand Kits and Knowledge Bases to maintain consistency and accuracy throughout the workflow.
Once your data is prepared, set up AI models to perform analysis, decision-making, or predictions. AirOps offers pre-designed templates or allows you to customize workflows for tasks like internal linking, alt tag optimization, or generating SEO content at scale. Each stage is interactive, providing opportunities to review, edit, and approve outputs.
Finally, AirOps ensures action execution is seamless with integrations into platforms like WordPress, Webflow, and Shopify. You can publish directly or export data for manual uploads, depending on your requirements.
With AirOps, building an AI workflow is no longer a technical hurdle but a streamlined process that delivers consistent, impactful results for your business.
Automate your process with AI to maximize your efficiency. Start building with AirOps today.
Closing Thoughts - Is Implementing an AI Workflow Worth It?
Implementing an AI workflow is undoubtedly worth it for businesses looking to improve efficiency, scalability, and precision in their operations. AI workflows automate repetitive tasks, enhance decision-making, and produce consistent results, freeing up teams to focus on strategic initiatives. Whether for content creation, SEO optimization, or data analysis, AI workflows streamline complex processes and deliver measurable outcomes.
If you’re ready to harness the power of AI workflows, AirOps offers the tools and flexibility to build workflows tailored to your goals. With intuitive design and robust integrations, AirOps makes implementing AI workflows seamless and impactful.
Scale your most ambitious SEO strategies
Use AI-powered workflows to turn your boldest content strategies into remarkable growth