What Are Three Types of Prompting in AI
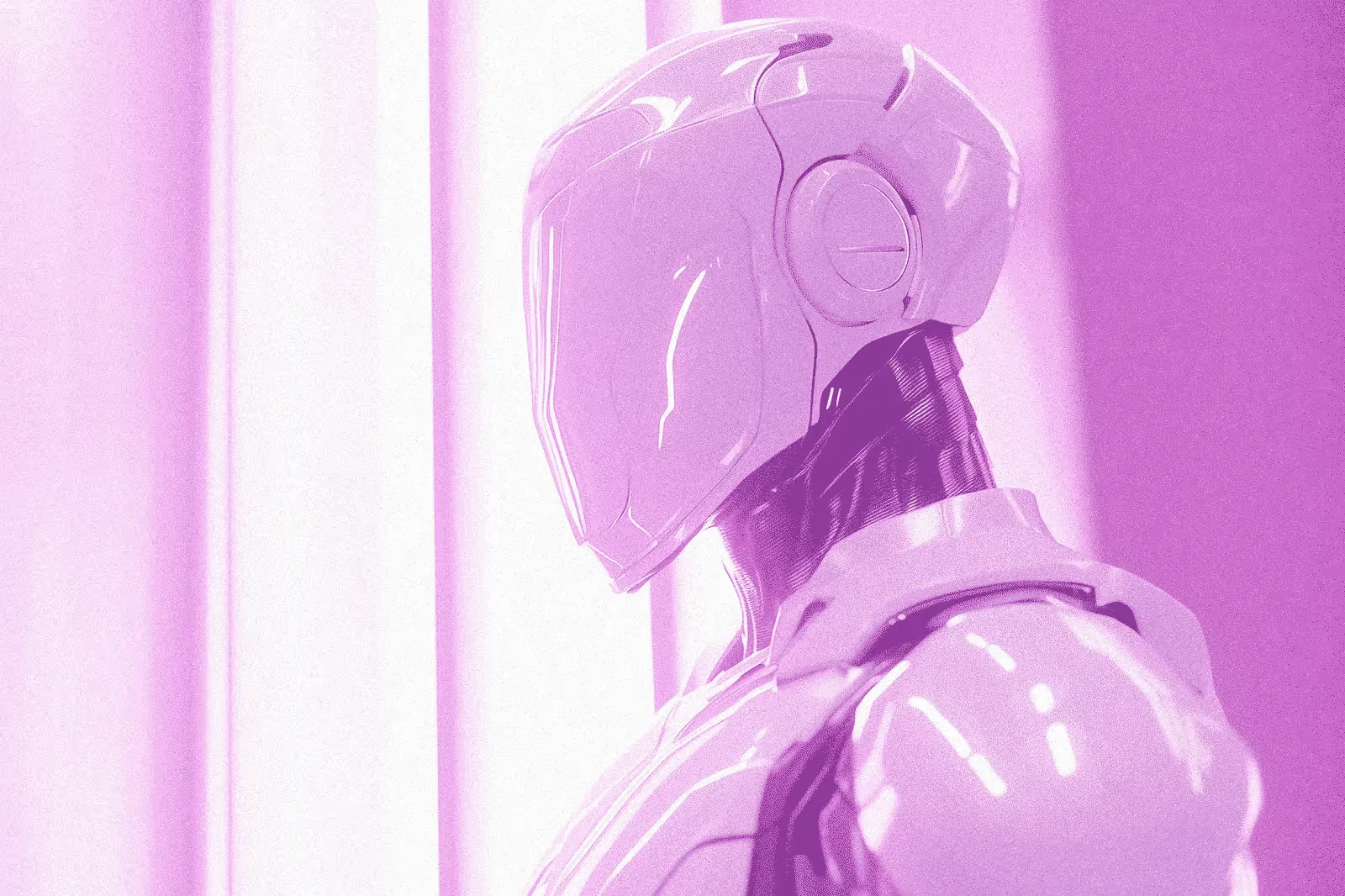
Have you ever wondered about the different types of prompting techniques used in artificial intelligence (AI)? Understanding these techniques can help you effectively interact with AI models and unlock their full potential.
In this article, we will explore three main types of prompting: N-shot prompting, Chain-of-thought (CoT) prompting, and Generated knowledge prompting. Each technique has its own strengths and applications in various AI tasks.
By the end of this article, you will have a clear understanding of how these prompting techniques work and when to use them to get the most out of AI models.
What are Three Types of Prompting in AI?
The three main types of prompting in AI are N-shot prompting, Chain-of-thought (CoT) prompting, and Generated knowledge prompting. Let's dive into each technique and explore their characteristics and applications.
N-shot Prompting
N-shot prompting involves providing the AI model with a series of input-output pairs to learn from. The model is exposed to multiple examples, allowing it to identify patterns and generalize its knowledge to new inputs.
This technique is particularly useful for specific tasks or domains where a limited number of examples are available. By leveraging N-shot prompting, AI models can quickly adapt to new tasks without requiring extensive training data.
Chain-of-thought (CoT) Prompting
Chain-of-thought prompting focuses on guiding the AI model's thought process by providing a sequence of prompts or questions. This technique encourages the model to think step-by-step and develop a coherent line of reasoning.
CoT prompting helps the model break down complex tasks into logical steps, leading to more accurate and contextually relevant responses. It is effective for tasks that require multi-step reasoning or problem-solving.
Generated Knowledge Prompting
Generated knowledge prompting leverages the AI model's existing knowledge to generate new information. This technique allows the model to expand its understanding and provide insights beyond the given input.
By prompting the model to generate knowledge, it can contribute to the development of novel ideas and solutions. Generated knowledge prompting is valuable for tasks that require creative thinking or the generation of new content.
Benefits of Prompting in AI
Prompting techniques offer several advantages that can significantly enhance the performance and capabilities of AI models.
One key benefit is the ability to improve model accuracy and generate more contextually relevant responses. By learning from carefully crafted prompts, AI models can better understand the nuances of the task at hand and provide more precise outputs.
Another advantage of prompting is enabling few-shot learning. This means that models can learn from a limited number of examples, reducing the need for extensive training data. Few-shot learning is particularly valuable when working with domain-specific tasks or when labeled data is scarce.
Moreover, prompting techniques expand the capabilities of AI models, allowing them to tackle complex reasoning tasks that might otherwise be challenging. By guiding the model's thought process through techniques like Chain-of-thought prompting, you can unlock the potential for generating novel ideas and solutions.
Prompting also enhances the model's ability to generalize knowledge to new inputs. By exposing the model to diverse examples and scenarios, it can learn patterns and apply its understanding to unseen data, improving its overall performance.
How does Prompting in AI Work?
Prompting techniques provide structured input to guide the model's output, ensuring that the generated content aligns with the desired task and context. N-shot prompting exposes the model to multiple input-output pairs, allowing it to learn patterns and generalize its knowledge to new inputs.
Chain-of-thought prompting breaks down complex tasks into logical steps, encouraging the model to think step-by-step and develop a coherent line of reasoning. This technique helps the model generate more accurate and contextually relevant responses.
Generated knowledge prompting leverages the model's existing knowledge to generate new insights. By prompting the model to expand its understanding, you can unlock its potential for creative thinking and the development of novel ideas.
The choice of prompting technique depends on the specific task requirements and available data. N-shot prompting is effective when working with limited examples, while Chain-of-thought prompting is valuable for tasks that require multi-step reasoning. Generated knowledge prompting is ideal for scenarios that demand creative thinking or the generation of new content.
What are the Challenges of Prompting in AI?
While prompting techniques offer numerous benefits, they also come with their own set of challenges. Understanding these challenges is important to effectively leverage prompting in AI applications.
Prompt Engineering Complexity
Crafting effective prompts requires careful consideration and experimentation. You need to strike a balance between specificity and generalization to ensure that the prompts guide the model towards the desired output without being overly restrictive. This process can be time-consuming and may require multiple iterations to achieve optimal results.
Dependence on High-quality Examples
The quality of the prompts directly impacts the model's performance. Curating diverse and representative examples is necessary for successful prompting. If the examples are biased, incomplete, or not representative of the task at hand, the model's output may be suboptimal or even misleading. Ensuring high-quality examples requires domain expertise and careful data selection.
Potential for Biased or Misleading Outputs
Prompts can inadvertently introduce biases or lead to misleading responses if not designed properly. The model may pick up on unintended patterns or generate outputs that are not aligned with the intended task. Careful prompt design and evaluation are necessary to mitigate these risks. You should regularly assess the model's outputs and iterate on the prompts to address any biases or inaccuracies.
Tips for Effective Prompting in AI
Provide Clear and Specific Instructions
To get the most out of AI models, it's important to provide clear and specific instructions in your prompts. Use precise language that guides the model towards the desired output. Include relevant keywords and specify the desired format for the generated content. This helps the model understand exactly what you're looking for and increases the likelihood of receiving accurate and relevant responses.
Leverage Iterative Refinement
Prompting is an iterative process. Engage in a conversation with the model by refining your prompts based on the initial responses you receive. If the output doesn't quite meet your expectations, adjust your prompts and try again. Experiment with different prompting techniques and variations to explore the model's capabilities and find the most effective approach for your specific task.
Incorporate Domain-specific Knowledge
Infusing your prompts with domain-specific knowledge can significantly enhance the model's understanding and performance. Utilize terminology and concepts specific to your domain when crafting prompts. This helps the model generate more accurate and contextually relevant responses. Leveraging your domain expertise in prompt design can lead to better results and more insightful outputs from the AI model.
Monitor and Evaluate Outputs
Regularly assess the quality and accuracy of the model's responses. Pay attention to the generated content and identify areas for improvement. If you notice biases, inaccuracies, or outputs that don't align with your expectations, adjust your prompts accordingly. Continuously monitoring and evaluating the model's performance allows you to refine your prompting techniques and ensure the AI system consistently delivers high-quality results.
How can Prompting Techniques Enhance Content Operations?
Prompting techniques have the power to revolutionize content operations, enabling you to generate high-quality content at scale. AI-powered prompts can automate content generation and optimization, streamlining your content creation process and saving valuable time and resources.
One notable example is how prompting techniques helped increase product page conversions by 18% through targeted and persuasive content. By leveraging AI-generated prompts, you can create compelling product descriptions that resonate with your target audience and drive conversions.
Moreover, prompting techniques have proven effective in boosting organic traffic by 20x in just 2 months. By generating SEO-friendly content at scale, you can significantly improve your search engine rankings and attract more organic visitors to your website.
Using AI to improve your SEO is another area where prompting techniques shine. AI-powered prompts can optimize your internal linking structure and backlink strategies, ensuring that your content is well-connected and authoritative in the eyes of search engines.
Prompting techniques find applications across various domains, including SEO content teams, e-commerce businesses, marketplace listings, and product teams. By leveraging AI-powered prompts, these teams can streamline their content creation and optimization processes, ultimately driving better results and enhancing overall content operations.
Understanding the three types of prompting in AI—N-shot prompting, Chain-of-thought (CoT) prompting, and Generated knowledge prompting—helps you effectively interact with AI models and enhance their performance in various tasks. By leveraging these techniques, you can improve model accuracy, enable few-shot learning, and tackle complex reasoning tasks.
AirOps can streamline your content creation and optimization processes by harnessing these AI prompting techniques, saving you time and resources while driving better results.
Win AI Search.
Increase brand visibility across AI search and Google with the only platform taking you from insights to action.
Get the latest on AI content & marketing
Get the latest in growth and AI workflows delivered to your inbox each week