Prompt Chaining vs. Chain of Thought
Table of Contents
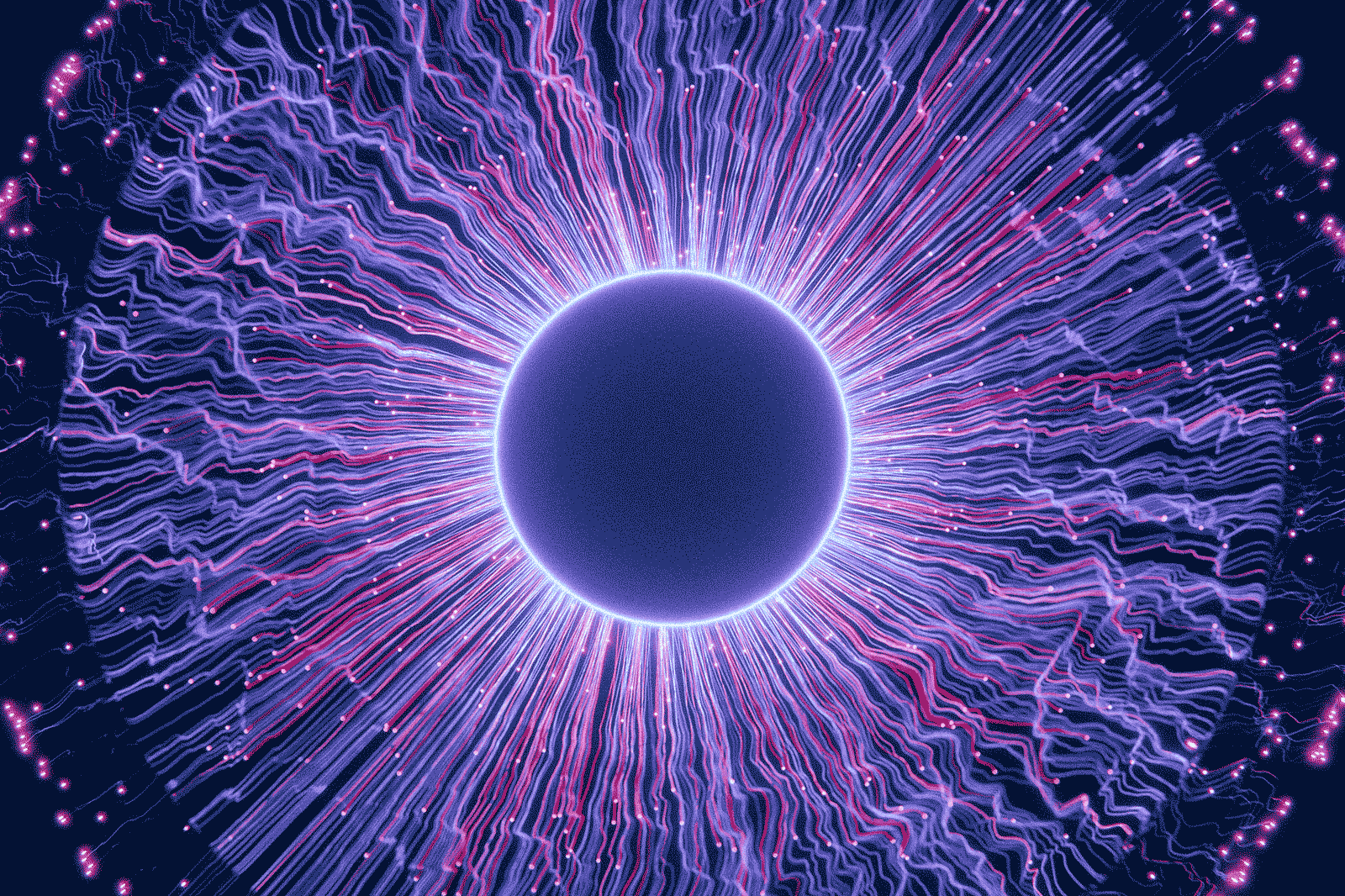
In the generative AI industry, prompt engineering plays a pivotal role in extracting accurate and meaningful outputs. Among the advanced techniques gaining traction are prompt chaining and chain of thought reasoning, two distinct methodologies that enhance the effectiveness of AI models. Both techniques involve structuring prompts to guide AI responses, but they differ significantly in approach and application.
Prompt chaining focuses on breaking complex tasks into a sequence of smaller, manageable steps, where each prompt builds on the output of the previous one. Chain of thought, on the other hand, encourages the AI to generate detailed, step-by-step reasoning within a single prompt to arrive at more accurate conclusions.
This article will discuss the core principles, use cases, and benefits of each technique, helping you determine when and how to apply them effectively to maximize the potential of generative AI.
Key Takeaways
- Prompt chaining breaks complex tasks into manageable steps, each addressed by a separate prompt, allowing for iterative refinement and higher-quality outputs.
- Chain of thought prompting encourages AI to process problems step-by-step within a single prompt, leading to cohesive reasoning and logically sound conclusions.
- Prompt chaining excels in multi-stage tasks, such as content creation and data analysis, while chain of thought shines in logical deduction and decision-making scenarios.
- Combining these techniques can maximize AI efficiency, improving content strategy, workflow management, and problem-solving outcomes.
- Tools like AirOps enhance both methods, providing structured workflows, centralized knowledge bases, and seamless AI integrations for optimal results.
What is Prompt Chaining?
Prompt chaining is a powerful technique that enhances content operations by breaking down larger tasks into a series of smaller, focused subtasks. Each subtask is addressed with its own prompt, allowing the AI model to concentrate on specific aspects of content generation and optimization one step at a time.
Example of Prompt Chaining
To illustrate how prompt chaining can be applied in content generation and optimization, consider the task of summarizing a long article. Here’s how you might effectively break down this process:
- Create an Initial Draft Summary: The first prompt instructs the model to read the article and generate a rough summary that captures the main points.
- Critique the Summary: The next prompt asks the model to evaluate the draft summary, identifying areas that require improvement or clarification.
- Check for Factual Inaccuracies: A third prompt directs the model to compare the summary against the original article, flagging any factual errors or inconsistencies.
- Produce a Refined Summary: The final prompt incorporates feedback from the critique and fact-checking steps, guiding the model to generate a polished and accurate summary.
By dedicating a separate prompt to each stage of the content creation process, you enable the AI model to focus fully on each subtask. This focused approach often results in higher-quality outputs compared to attempting to tackle the entire task with a single prompt. Utilizing prompt chaining not only improves content generation but also enhances the overall optimization of your content strategy.
Benefits of Prompt Chaining
Implementing prompt chaining in your content strategy can significantly enhance the quality and efficiency of AI-driven projects. Here are the main benefits prompt chaining provides:
- Increased Focus on Subtasks: By breaking down complex tasks into smaller subtasks, prompt chaining allows the AI model to focus on each step, resulting in higher-quality outputs that align with your content strategy.
- Easier Collaboration and Understanding: Prompt chaining enhances team collaboration by clarifying each step of the AI-driven process, making it easier for team members to contribute their expertise and for stakeholders to grasp the workflow.
- Applicability Across Domains: This versatile technique improves output quality across various tasks, from generating content to optimizing product descriptions, leading to more accurate and coherent results.
- Improved Efficiency and Scalability: Prompt chaining streamlines content creation, enabling faster and more scalable production without sacrificing quality—an essential advantage for businesses aiming to grow their content efforts.
- Enhanced Troubleshooting and Refinement: With prompt chaining, identifying and addressing issues in AI-generated content becomes simpler. This targeted approach allows for efficient refinement of specific subtasks, saving time and resources in the optimization process.
What is Chain of Thought Prompting?
Chain of thought prompting is a technique used for enhancing content operations, particularly in the areas of content generation and optimization. Rather than breaking a task into smaller subtasks like prompt chaining, this method encourages the AI model to think through a problem step-by-step within a single prompt, facilitating deeper reasoning and more coherent outputs.
Example of Chain of Thought Prompting
To illustrate how chain of thought prompting can be applied in content generation and optimization, consider the task of creating a detailed article outline. Here’s how you can guide the AI model through this process:
- Identify the Main Topic: Start by prompting the model to define the central theme or subject of the article, ensuring clarity on what needs to be addressed.
- List Key Points: Next, ask the model to brainstorm and list key points or subtopics that should be covered in relation to the main topic.
- Organize the Structure: Prompt the model to arrange these key points into a logical structure, outlining sections and subsections for the article.
- Refine with Details: Finally, instruct the model to elaborate on each section with specific details, examples, or data that will enrich the content.
By guiding the AI through this step-by-step reasoning process, you encourage it to produce a well-organized and comprehensive outline. This approach not only improves the quality of content generation but also enhances optimization by ensuring that all relevant aspects are considered and logically presented. Chain of thought prompting can be effectively applied across various content tasks, from drafting articles to developing marketing strategies, leading to more thoughtful and high-quality outputs.
Benefits of Chain of Thought Prompting
Utilizing chain of thought prompting in your content strategy can greatly enhance the depth and clarity of AI-generated outputs.
- Enhanced Logical Reasoning: Chain of thought prompting encourages the AI model to engage in structured reasoning, which helps produce more coherent and logically sound content. This is particularly valuable for tasks that require analytical thinking or problem-solving.
- Improved Content Quality: This technique fosters deeper insights and more nuanced outputs by guiding the model through a step-by-step reasoning process, ultimately leading to higher-quality content that resonates with your audience.
- Greater Clarity and Focus: This approach allows for clearer articulation of ideas, as it breaks down complex concepts into manageable steps. The result is content that is easier for readers to understand and follow.
- Effective Decision-Making: Chain of thought prompting is especially useful in scenarios that involve strategic decision-making. By systematically evaluating different factors, the AI can provide well-supported recommendations that align with your content goals.
- Streamlined Content Development: Incorporating chain of thought prompting into your workflow can streamline the content development process, making it easier to generate comprehensive articles, reports, or analyses that require a thorough exploration of a topic.
Key Differences Between Prompt Chaining and Chain of Thought Prompting
Both prompt chaining and chain of thought prompting enhance the capabilities of generative AI, but their methodologies and applications vary significantly. Understanding these differences can help determine which approach is better suited for specific tasks.
Focus and Process
Prompt chaining emphasizes breaking complex tasks into smaller, manageable subtasks, each handled through a separate prompt. This modular approach allows the AI to focus exclusively on one aspect of a task at a time, ensuring precision and clarity. For example, in content creation, this could involve creating an outline first, drafting individual sections, and refining each part based on feedback. The step-by-step nature of prompt chaining also makes it highly effective for tasks like data analysis, where incremental refinement is essential.
In contrast, chain of thought prompting encourages the AI to think through a task in its entirety within a single prompt. This method is particularly suited for tasks requiring logical deduction or strategic reasoning. For instance, when analyzing audience insights or developing a content strategy, chain of thought prompting helps the AI evaluate multiple factors cohesively and arrive at well-supported conclusions.
Prompt Structure
Another distinction lies in how prompts are structured.
- Prompt Chaining: Each subtask is given its own dedicated prompt. This separation ensures the AI concentrates on specific details, making it ideal for multi-step processes where outputs are reviewed and refined iteratively.
- Chain of Thought Prompting: A single, detailed prompt guides the AI through the entire reasoning process. This approach fosters a holistic and interconnected understanding of the task, enabling comprehensive and logical outputs.
Ideal Use Cases
- Prompt Chaining: Best suited for content creation tasks such as drafting articles, generating product descriptions, and iterative learning processes like debugging code.
- Chain of Thought Prompting: Excels in scenarios requiring logical reasoning, decision-making processes, and multi-step analyses related to content strategy.
When deciding between these two techniques, consider the nature of your task. If it involves multiple stages or benefits from iterative refinement, prompt chaining is likely the better choice.
Conversely, if your task requires structured reasoning or complex problem-solving, chain of thought prompting can guide the model to produce more accurate and coherent outputs. Both techniques can significantly enhance the quality and effectiveness of AI-generated content when applied strategically.
How to Implement Prompt Chaining and Chain of Thought Prompting in Your Content Strategy
Integrating both prompt chaining and chain of thought prompting into your content strategy can significantly enhance the quality and efficiency of AI-driven content generation. Here’s how to implement these techniques effectively:
1. Break Down the Problem into Subtasks
Start by dividing your content creation task into clear, sequential subtasks. Each step should focus on a specific aspect of the project, forming the foundation for your prompt chain.
For example, if you’re creating a blog post, you might break it down into the following subtasks:
- Research the topic and gather relevant information.
- Create an outline for the post.
- Write the introduction.
- Develop the main points.
- Craft the conclusion.
- Edit and refine the post.
2. Plan the Handoff Between Prompts
To ensure a seamless transition between prompts in your chain, carefully plan how each output will feed into the next input. Here are some tips for effective handoffs:
- Focus on Relevant Information: Ensure that each prompt's output includes only the information necessary for the next step in the chain.
- Map Output to Input: Structure the output from one prompt so it can be easily utilized as input for the subsequent prompt.
- Avoid Information Overload: Keep outputs concise and focused to prevent confusion or errors in later prompts.
3. Incorporate Chain of Thought Prompting
While implementing prompt chaining, consider using chain of thought prompting within each subtask to encourage deeper reasoning and analysis. For instance, when developing main points, guide the AI through logical steps that explore different angles or supporting arguments.
4. Rapidly Experiment and Iterate
The implementation process requires rapid experimentation and iteration. Test various prompt variations, tweak your chain structure, and adjust output scopes to refine results effectively. Here are strategies for successful experimentation:
- Test Prompt Variations: Experiment with different phrasings and examples to optimize each prompt in the chain.
- Tweak the Chain Structure: If outputs aren’t meeting expectations, consider adjusting the sequence or adding steps to enhance clarity.
- Adjust Output Scope: Modify what you ask for in each prompt to ensure you receive the appropriate level of detail and specificity.
5. Use Tools that Naturally Use Both Prompting Techniques
Leveraging tools that seamlessly integrate both prompt chaining and chain of thought prompting can significantly enhance the efficiency and quality of your outputs. Such tools are designed to adapt to the specific needs of each task, whether it requires breaking down complex workflows into manageable steps or solving intricate problems with cohesive reasoning.
AirOps is uniquely positioned to support both techniques, offering advanced features that streamline content creation and problem-solving:
- Grids for Workflow Management: With AirOps Grids, teams can break tasks into individual steps, enabling prompt chaining for projects like content creation, SEO optimization, or multi-stage analysis. This ensures every step is precise and builds on the previous one.
- Knowledge Bases for Strategic Reasoning: By centralizing brand knowledge, AirOps helps maintain consistency and depth in reasoning processes. This is ideal for chain of thought prompting, allowing the AI to draw on accurate data and context when solving complex problems.
- Access to Multiple AI Models: AirOps integrates with over 40 AI models, offering the flexibility to apply the most effective techniques for specific tasks. Whether it’s generating detailed content through iterative prompts or fostering logical reasoning in a single query, AirOps ensures optimal performance.
- Seamless Integration: The platform connects with CMSs, data warehouses, and SEO tools, enabling the smooth execution of both chaining and reasoning processes across diverse applications.
Ready to see how the best in AI automation can help your processes? Start building smarter with AirOps today.
Challenges and Considerations in Prompt Chaining
While prompt chaining offers numerous benefits for enhancing content strategy, it's important to be aware of potential challenges when implementing this technique.
Increased Complexity and Potential for Errors
Prompt chaining involves multiple prompts in the workflow, which can increase the overall complexity of the system. This complexity may lead to a higher risk of errors, such as misaligned outputs or loss of context between steps.
To mitigate these risks, carefully plan the handoff between prompts, ensuring that each output aligns with the input requirements of the next prompt. Regularly monitoring performance and being prepared to troubleshoot can help maintain the quality of your content.
Higher Costs and Processing Time
Each prompt in a chain requires its own API call, which can result in higher costs compared to using a single prompt. This is especially true if the total input tokens across the chain exceed those used in a more straightforward approach.
Additionally, processing time for a prompt chain is likely to be longer since the model generates outputs for each step in the sequence. To manage costs and time, consider optimizing the number of prompts and their scope while leveraging tools like AirOps that streamline this process.
Balancing Benefits and Drawbacks
Despite these challenges, the potential gains in output quality and coherence often outweigh the drawbacks of prompt chaining. By breaking down complex tasks into smaller, manageable subtasks, you enable AI models to focus on one aspect at a time, resulting in higher-quality and more coherent content. This technique aligns perfectly with goals such as achieving impactful content at scale.
Closing Thoughts - Is Prompt Chaining Worth Implementing in Your AI Workflow?
Yes, prompt chaining is a valuable technique for AI workflows, particularly for tasks requiring structure, precision, and iterative refinement. By breaking complex projects into smaller, manageable steps, prompt chaining ensures that each stage of the process receives focused attention. This approach not only improves the quality of outputs but also enhances efficiency, making it ideal for workflows like content creation, data analysis, and technical problem-solving.
When integrated with tools designed to optimize and streamline these processes, prompt chaining becomes even more impactful. Platforms like AirOps simplify the execution of prompt chaining by offering features such as Grids for managing workflows, Knowledge Bases for centralized expertise, and access to leading AI models for diverse applications.
Prompt chaining is a technique worth adopting for businesses aiming to unlock the full potential of generative AI.
Ready to take your AI workflows to the next level with powerful tools and strategies? Start building smarter with AirOps today.
Scale your most ambitious SEO strategies
Use AI-powered workflows to turn your boldest content strategies into remarkable growth