Gen. AI vs. LLM: Key Differences & Similarities
Table of Contents
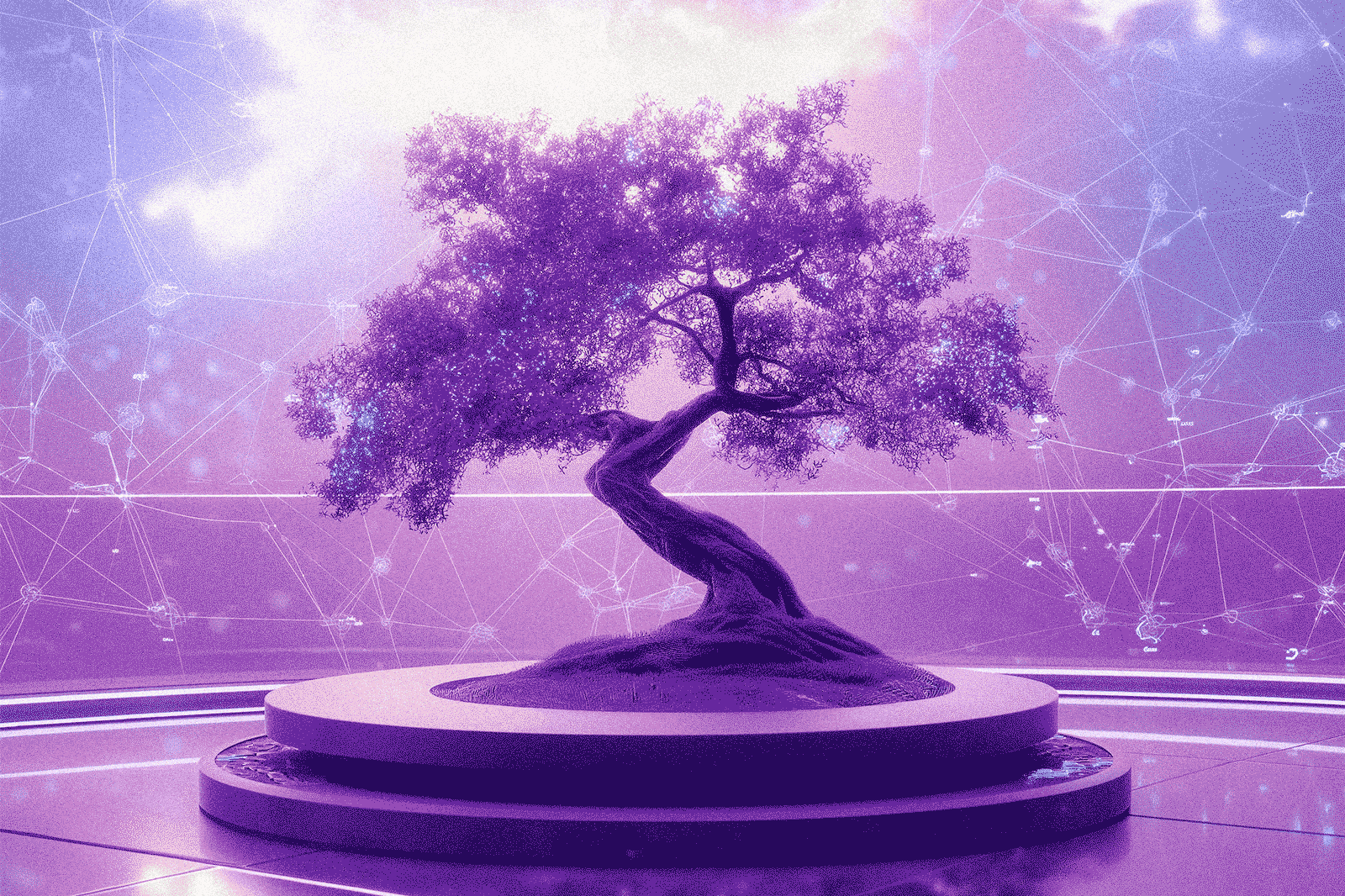
If you’ve ever read articles on AI, you’ve most likely read the words generative AI and LLMs thrown around here and there.
Both these terms are pivotal in shaping how machines create and interpret information, yet they are often confused or used interchangeably. While both fall under the umbrella of artificial intelligence, there are some key differences between the two that are important to understand.
In this article, we'll talk about generative AI and LLMs, uncovering their unique characteristics, applications, and how they work together to revolutionize various industries.
What is Generative AI?
Generative AI is a broad category of AI systems that can create new, original content based on patterns learned from existing data. These systems use advanced machine learning techniques to analyze and understand the underlying structure and characteristics of the input data, allowing them to generate novel outputs that resemble the original content but with a touch of creativity to create something brand new.
The power of generative AI lies in its ability to produce a wide range of content types, from text and images to music and 3D models.
By training on vast datasets, generative AI models can capture the essence of the input data and create new, unique content that feels authentic and contextually relevant.
Applications of Generative AI
Text generation is one of the most common applications of generative AI. Models like GPT-4 can produce coherent, human-like text based on a given prompt or context. This has significant implications for content creation, chatbots, and language translation.
Image creation is another exciting area where generative AI shines. Tools like DALL-E and Midjourney can generate stunning, original images based on textual descriptions, opening up new possibilities for artists, designers, and marketers.
In the realm of music, generative AI models can compose unique pieces based on the styles and patterns of existing compositions. This technology has the potential to revolutionize the music industry, enabling musicians to explore new creative avenues and generate original content more efficiently.
Video synthesis is an emerging application of generative AI, allowing for the creation of realistic video content based on learned patterns and characteristics. This has implications for the entertainment industry, as well as for training and educational purposes.
What are Large Language Models (LLMs)?
LLMs are a specific subset of generative AI that focus on processing and generating human-like text. They are trained on massive amounts of textual data, allowing them to understand and generate coherent, contextually relevant language.
The training process involves feeding the model a vast corpus of text data, such as books, articles, and websites. Through this process, LLMs learn the intricacies of language, including grammar, syntax, and semantics. They also develop an understanding of context and relationships between words and concepts.
Once trained, LLMs can perform a wide range of language-related tasks, such as text generation, language translation, sentiment analysis, and question answering. They can generate coherent and fluent text that closely resembles human writing, making them valuable tools for various applications.
Examples of LLMs
One of the most well-known LLMs is the GPT (Generative Pre-trained Transformer) series developed by OpenAI. GPT models, such as GPT-4, have demonstrated remarkable capabilities in generating human-like text, completing tasks like writing articles, answering questions, and even coding.
Another prominent LLM is BERT (Bidirectional Encoder Representations from Transformers), developed by Google. BERT is designed to understand the context and meaning of words in a sentence by considering both the left and right context. This bidirectional approach enables BERT to perform well on tasks like sentiment analysis and named entity recognition.
T5 (Text-to-Text Transfer Transformer) is an LLM developed by Google that can perform a wide range of language tasks, including translation, summarization, and question-answering. T5 is trained on a diverse dataset and can generate high-quality text outputs for various applications.
These are just a few examples of the many LLMs available today. As research in this field continues to advance, we can expect to see even more powerful and versatile LLMs in the future, opening up new possibilities for natural language processing and generation.
Key Differences Between Generative AI and LLMs
While generative AI and LLMs share the common goal of creating new content, they differ in several key aspects that shape their applications and impact across various domains.
Scope
Generative AI encompasses a broad spectrum of AI technologies dedicated to producing new content across diverse formats, including text, images, music, and code. This wide scope allows generative AI to cater to a variety of industries and use cases, from creative arts to scientific research.
In contrast, LLMs are a specific subset of generative AI that focuses solely on processing and generating human-like text. Their specialized nature makes them particularly well-suited for tasks involving natural language understanding, generation, and manipulation.
Output
The output of generative AI models can take many forms, depending on the specific application and training data. These models can create images, videos, audio, 3D models, and even code, showcasing the versatility and potential of generative AI in various domains.
LLMs, on the other hand, primarily generate text-based outputs. While this may seem limited compared to the diverse outputs of generative AI, the ability to produce coherent, contextually relevant text is invaluable for a wide range of applications, from content creation to chatbots and language translation.
Training Data
Generative AI models can be trained on various data types, depending on the desired output. For example, a generative AI model designed to create images would be trained on a dataset of images, while a model focused on music generation would learn from a corpus of musical compositions. This flexibility in training data allows generative AI to adapt to different domains and create content that aligns with the characteristics of the input data.
LLMs, being focused on text generation, are trained exclusively on large volumes of textual data. This specialized training enables them to capture the nuances and complexities of human language, allowing them to generate fluent and contextually appropriate text.
Applications
The broad scope and diverse outputs of generative AI make it applicable across a wide range of industries. In creative fields like art, music, and design, generative AI can be used to create new and unique content, assisting artists and designers in their creative processes. In scientific research, generative AI can aid in data augmentation, creating synthetic datasets for training other AI models. Generative AI also has applications in gaming, where it can be used to generate new levels, characters, or even entire virtual worlds.
LLMs, with their focus on language-related tasks, are particularly well-suited for applications in natural language processing. They can be used for content creation, generating articles, product descriptions, and even poetry. LLMs also power chatbots and virtual assistants, enabling more natural and engaging conversations with users. In the realm of language translation, LLMs can help break down language barriers by providing accurate and context-aware translations.
How Do Generative AI and LLMs Work Together?
While generative AI and LLMs have distinct characteristics and applications, they can also work together in powerful ways to enhance various AI-driven solutions.
DALL-E is a perfect example. When you input a text prompt into DALL-E, the LLM component first interprets the language. It deciphers the meaning, context, and intricacies of your prompt, transforming the text into an internal conceptual representation. This is where the LLM’s deep language comprehension comes into play, ensuring that the prompt is accurately understood.
Once the text is interpreted, the Generative AI aspect takes over. Using the internal representation created by the LLM, the Generative AI model synthesizes a new image that visually captures the concept described. This collaboration between LLMs and Generative AI allows for the seamless creation of complex, detailed images from mere text, showcasing how these two technologies complement each other to generate innovative content.
Applications of Generative AI and LLMs
Generative AI and LLMs have the potential to revolutionize various industries and transform the way you interact with technology. Let's explore some of the most exciting applications of these cutting-edge technologies.
Content Generation
Generative AI and LLMs are revolutionizing content creation by producing high-ranking, audience-focused articles that balance SEO expertise with a human touch. These technologies allow you to automate and scale content production while maintaining quality, authenticity, and brand consistency. You can easily integrate first-party and third-party data into your content, ensuring it resonates with your audience.
With tools for blending AI-generated visuals, automating workflows with human review, and leveraging your content library, you can scale content without sacrificing quality. These solutions enable you to write on-brand, long-tail content, optimize it for SEO with advanced keyword strategies, and automate technical SEO tasks—all while driving organic traffic and boosting search visibility.
In fact, Deepgram, a leading provider of speech recognition technology, was able to boost their organic traffic by 20x in just 2 months by leveraging AI-generated content. This demonstrates the power of these technologies in driving real-world results.
Personalization and Recommendations
Another application of generative AI and LLMs is personalization and recommendations. These technologies can analyze vast amounts of user data to generate customized content that aligns with individual preferences and interests.
E-commerce platforms are greatly implementing AI to automatically generate personalized product descriptions and recommendations based on your browsing and purchase history.
Rare Candy, a collectibles marketplace, was able to increase their product page conversions by 18% by leveraging AI-powered personalization. This highlights the potential of these technologies in driving engagement and conversions.
Chatbots and Virtual Assistants
Generative AI and LLMs are also transforming the way you interact with chatbots and virtual assistants. These technologies enable more natural, interactive conversations that feel more human-like and engaging.
Imagine having a virtual assistant that can not only understand your queries but also provide contextually relevant and personalized responses. Or a chatbot that can handle complex customer service inquiries with ease, providing accurate and helpful information.
ChatGPT, one of the most advanced language models available today, has demonstrated the ability to generate answers to a wide range of user queries. This showcases the potential of these technologies in enhancing conversational AI experiences.
Multimodal Content Generation
Generative AI and LLMs are not limited to generating content in a single modality. These technologies can also work together to create richer, more immersive experiences by combining language understanding with content generation across multiple modalities.
Midjourney, a popular AI image generation tool, has shown the potential of these technologies in creating photorealistic results based on textual prompts. This demonstrates the power of multimodal content generation in pushing the boundaries of what's possible with AI.
Choosing the Right Approach for Your Business
With AI, there isn’t a one-size-fits-all approach to optimizing your processes. What works for one company won’t necessarily work for another. Before integrating AI in your workflow, consider these core aspects that are going to influence your decision.
Type of Content
When deciding between generative AI and LLMs for your business, consider the type of content you need to generate and the data available to train your models. If you require a diverse range of content types, such as images, music, or 3D models, generative AI may be the better choice. However, if your focus is primarily on text-based content, LLMs are likely the most suitable option.
Complexity and Scalability
Evaluating the complexity of your tasks and scalability requirements is also important. Generative AI excels at handling complex, creative tasks that require diverse outputs, while LLMs are well-suited for language-related tasks that demand accurate and coherent text generation.
Availability of Technical Expertise
The level of development expertise within your organization and the ethical considerations surrounding your AI implementation should also factor into your decision. Developing and fine-tuning generative AI models often requires specialized knowledge and resources, whereas LLMs, particularly pre-trained models, may be more accessible and user-friendly for teams with less AI experience.
Ultimately, the choice between generative AI and LLMs depends on your specific business needs, resources, and goals. Carefully assessing these factors will help you determine the most appropriate approach for your organization, ensuring that you harness the power of AI effectively and responsibly.
Navigating the Ethical Challenges of Generative AI and LLMs
As you explore the potential of generative AI and LLMs, it's important to be aware of the ethical challenges that come with these powerful technologies. Addressing these challenges head-on will help ensure that you use these tools responsibly and effectively.
Biased Training Data
One key concern is the potential for biases in the training data used to develop generative AI and LLM models. Generally, AI is not biased. However, if the training data contains inherent biases, such as gender or racial stereotypes, these biases can be amplified and perpetuated in the generated content. To mitigate this risk, you should carefully curate and preprocess your training data, ensuring that it is diverse, representative, and free from harmful biases.
Authenticity
Another important consideration is ensuring responsible use and content authenticity. Generative AI and LLMs can be used to create highly realistic content that may be difficult to distinguish from human-created work. This raises questions about the potential for misuse, such as generating fake news, impersonating individuals, or engaging in plagiarism.
To address these concerns, you should establish clear guidelines and policies for the use of these technologies, and implement mechanisms for content verification and attribution.
Plagiarism
Plagiarism is another critical ethical challenge when using Generative AI and LLMs. These technologies have the power to produce content that closely resembles existing works, sometimes even unintentionally replicating phrases or ideas from their training data.
This can lead to issues of intellectual property infringement or accidental plagiarism, where generated content may not be entirely original. To avoid this, it's essential to implement rigorous checks to ensure the originality of the output. Using plagiarism detection tools, properly attributing sources, and setting clear boundaries for acceptable content generation are key steps in maintaining ethical standards.
As you continue to explore the possibilities of generative AI and LLMs, keep these ethical considerations at the forefront of your mind. By proactively addressing potential biases, ensuring responsible use, and maintaining content authenticity, you can harness the power of these technologies in a way that is both effective and ethical.
Closing Thoughts - The Endless Potential of Generative AI and LLMs
Generative AI and LLMs have the potential to practically reinvent business operations by addressing key pain points such as content creation, customer engagement, and data analysis. These technologies can automate repetitive tasks, reduce operational costs, and enhance creativity, allowing businesses to focus on strategic decision-making. Moreover, their ability to generate personalized content at scale can improve customer experiences, driving greater engagement and loyalty.
However, the integration of these tools also presents challenges, such as maintaining quality control and avoiding ethical pitfalls like plagiarism. Businesses that effectively solve these challenges stand to gain a competitive edge, leveraging AI to innovate and streamline their workflows.
If you're looking for an AI workflow tool to enhance your business operations, AirOps is one of the best platforms you can work with. AirOps offers a comprehensive platform designed to help businesses build, manage, and scale AI-driven workflows with ease. Whether you're improving content creation, streamlining customer interactions, or integrating AI into existing systems, AirOps delivers powerful tools tailored to your needs.
With features like integrating first-party and third-party data, automating workflows with human review, and preserving brand consistency, AirOps ensures that your AI-driven operations maintain quality and authenticity. The platform supports over 40 AI models, including GPT-4 and Claude 2, allowing you to create and optimize AI prompts, manage data inputs, and deploy workflows at scale.
Additionally, AirOps enhances SEO by allowing you to implement custom, programmatic strategies, automate technical SEO tasks, and build deep topical authority. It also provides multimodal inputs (text, video, audio) and workflow steps that include human review and output evaluation. Whether in e-commerce, content marketing, or product development, AirOps helps transform visionary ideas into actionable workflows, driving real business results with enterprise-grade security and compliance.
Sign up and start driving organic growth today with AirOps today!
Scale your most ambitious SEO strategies
Use AI-powered workflows to turn your boldest content strategies into remarkable growth